SentioCX announces the launch of SentioManager v2
Ronald Rubens
Enhancing Human-In-The-Loop orchestration with capabilities such as Sentiment Analysis
by: Ronald Rubens
Amsterdam, May 28th,2024 – SentioCX, a leader in AI-enabled Conversation Orchestration, proudly announces the release of SentioManager v2. This new version introduces groundbreaking capabilities designed to optimize and transform customer interactions, providing enterprises and our partners with cutting-edge tools to elevate their customer experience strategies.
Key Features and Enhancements in SentioManager v2:
1. Intent-Based Predictive Routing with Sentiment Analysis: SentioManager v2 now includes sentiment analysis to enhance intent-based routing. The continuous triaging algorithm ranks interactions not only based on the importance and urgency of the intent, but also allows the sentiment to dynamically adjust prioritization. This ensures that interactions with positive or negative sentiments are handled appropriately with the right priority and the best-matched agent, improving customer satisfaction and engagement.
2. SentioSimulator for predictive traffic simulation: The new SentioSimulator feature allows users to simulate incoming traffic and predict the effectiveness of the intent-based routing algorithm. This capability is also pivotal for automating SLA (Service Level Agreement) management, ensuring that routing adjustments are self-regulating and optimized for incoming traffic.
3. Advanced SLA Insights: SentioManager v2 provides comprehensive insights into SLA attainment, offering users an immediate overview of SLA management effectiveness. This feature highlights the impact of customer interactions on key performance indicators (KPIs) such as customer churn, Net Promoter Scores (NPS), customer satisfaction (CSAT), and sales metrics.
4. Seamless Integration with BYOB (Bring Your Own Bot): In our commitment to preventing ecosystem lock-in by existing CCaaS and CEC players, we continue to support various conversational AI platforms. With the release of SentioManager v2, we are excited to introduce our enhanced Seamless Hand-off API. This API, now publicly available on our development hub (https://developers.sentio.cx/welcome.html), ensures smooth integration with your conversational AI platform.
5. Human-In-The-Loop Orchestrator for RPA (Robotic Process Automation): SentioManager v2 includes a Human-In-The-Loop orchestrator, which is crucial for processes requiring real-time human intervention. This feature prioritizes tasks based on the importance or urgency of intent and/or sentiment, and matches them real-time with the right experts possessing the necessary skills and proficiency levels.
“We are excited to launch SentioManager v2, a significant upgrade that underscores our commitment to making a positive impact on our customers and partners,” said Ronald Rubens, founder & CEO at SentioCX. “These new capabilities empower enterprises to refine their customer experience strategies with effortless transitions from bots, automated SLA management, advanced analytics, predictive simulations, and seamless integrations.”
Adrian Ivan, CTO at SentioCX, added, “SentioManager V2 reinforces our commitment to delivering exceptional performance while upholding stringent security and privacy standards. We are dedicated to providing a reliable and secure platform that best supports our customers.”
For more information about SentioManager v2 and how it can transform your customer and employee experiences, please contact us at info@sentiocx.com
About SentioCX: SentioCX is a leading provider of AI-enabled Conversation Orchestration, dedicated to helping businesses improve customer interactions through innovative technology and data-driven insights. Our solutions enable companies to enhance customer satisfaction, sales, loyalty, and operational efficiency.
For more information, please contact:
info@sentiocx.com
+31655884733
SentioCX B.V.
Barbara Strozzilaan 101
1083 HN Amsterdam, the Netherlands
How to Automate SLA Management in contact centers to meet a new 3-minutes-law?
The Spanish Council of Ministers recently introduced a new regulation, known as the “the 3-minutes law”. This new law requires public and private companies in utilities, transport, postal services, communications, and financial services, as well as companies with over 250 employees or an annual turnover exceeding 50 million euros, to meet quality standards in customer service. The most important one is that calls (or chats) need to be answered within 3 minutes by live agents – especially when customers get stuck in automated services such as bots or IVRs. If these public or private companies do not comply with the 3 minutes law, they can be fined up to €100,000 for breaches. Consumer Rights Minister Alberto Garzon said in a new conference:
“The practically infinite waiting times that produce frustration are over"
Subsequently, the Spanish press argued that it’s more essential than ever for contact centers to not only ensure adequate staffing but also to monitor compliance. The question in this blog is: how we can comply to these new standards, without significantly increasing costs by adding more agents and supervisors to monitor SLA’s.
Current challenges
Let’s explore two significant challenges in today’s contact centers related to SLA management.
1. 62% of transitions from Self-Service to Agent Service are ‘High Effort’
When agents are busy, and customers expect a handoff to a live agent, they often face unpredictable wait times and poor first-time resolution. In addition to exposure to heavy fines and reputational damage in countries like Spain, the net effect is a significant impact on KPIs such as customer churn, customer satisfaction, and sales. The transitions are simply not seamless enough.
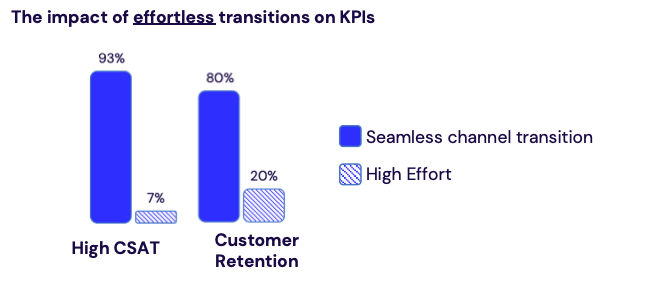
Modern orchestration requires context about intents and circumstances to guide the experience and understanding of the relative importance and urgency for both the user/customer and the business.
2. Traditional Queuing Requires Manual SLA Management
During our work on the Sentio approach to orchestration, I traveled to New York JFK Airport and experienced firsthand why manual SLA management is still necessary, even for two simple queues. This reminded me of the current state of technology in contact centers.
At JFK Airport, the SkyTeam lounge was at full capacity. To manage access, two queues were created: one for business-class passengers and another for SkyPriority passengers with lounge access eligibility. Unsurprisingly, business-class passengers received the highest priority. SkyTeam assigned a ‘traffic police officer’ to manage the queues at the lounge entrance.
What followed was predictable: a retired couple in the regular queue became upset after waiting for 45 minutes, while business-class passengers only waited for 10 seconds. Only when they emphasized their 30-year loyalty to SkyTeam did they receive priority access. The SkyTeam ‘traffic police officer’ was visibly stressed and unable to handle the complaints, eventually needing her manager to intervene.
This example illustrates a simple truth: queues complicate operational management through manual reordering and shifting of interactions, exploiting manual SLA loopholes. This problem grows as the number of queues increases due to the need to pair agent profiles, skills, and proficiency with specific customer needs, or to create priority queues for different requirements (e.g., a French-speaking airline reservation customer service). This explosion in queues is depicted in the diagram below:
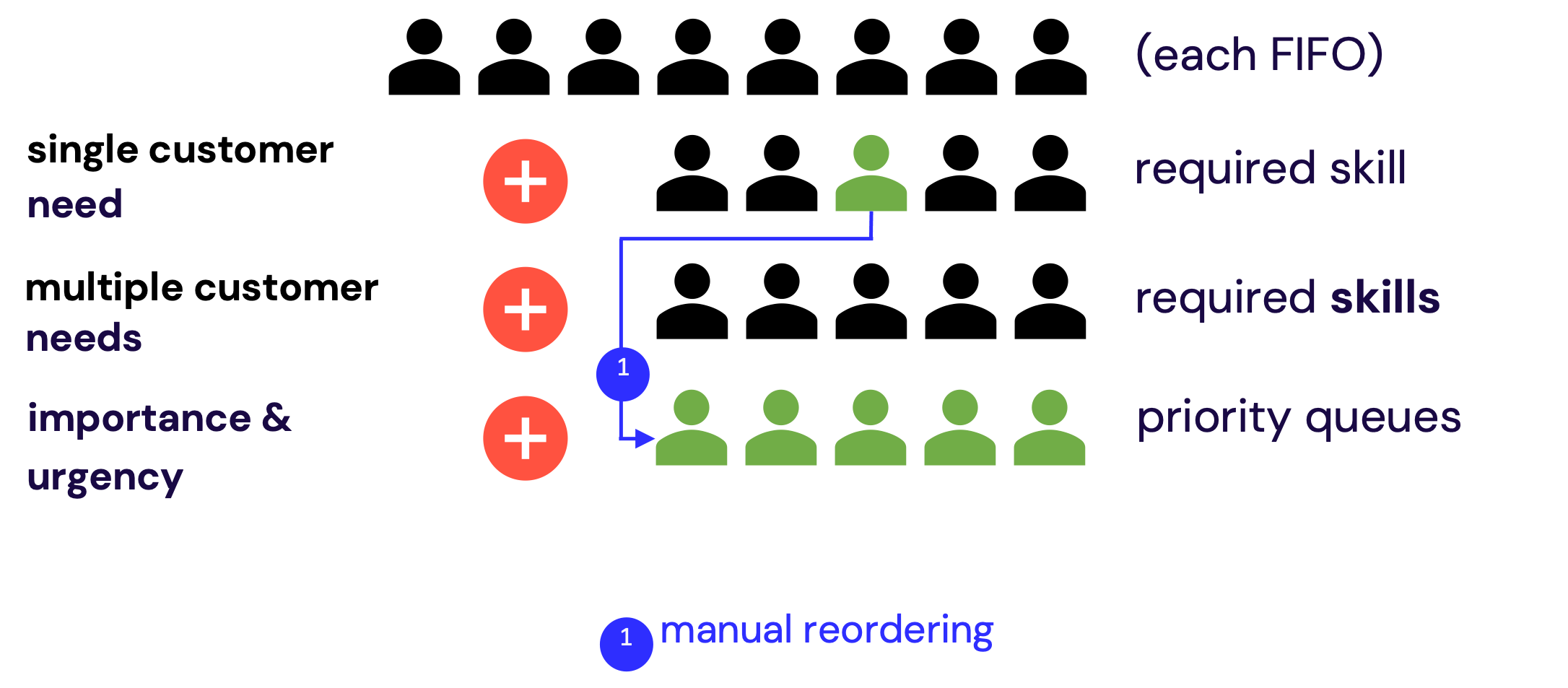
The result is an increase in operational costs and the need for supervisor intervention.
How can we overcome these Challenges?
a. Understanding what makes Hand-Offs High-Effort’ by Implementing a Seamless Hand-off protocol between Self-Service and the Contact Center, CEC or RPA platform
Integrating conversational AI/bot platforms with CX platforms should not be done by merely throwing conversations over the fence. Instead, it is much more effective to gain advanced insights and predictive actions on agent availability, required skills, and proficiency levels. As the ‘native intent’ in the conversational AI or bot platform reveals the ‘real issue or need,’ these intents should be classified and recognized for triaging and pairing purposes.
Secondly, instead of having people stuck in queues without visibility of wait times, continuous engagement should be facilitated with the bot, including announcements of predictive wait times and escalation of priority based on an evolving conditions or sentiment. In other words, when people show emotion or reveal signs of dissatisfaction, real-time and continuous triaging should be used to adjust the priority of getting connected with the right relevant live agent.
Thirdly, the most impactful conversations should be prioritized through triaging to optimize business outcomes. For example, a person who wants to cancel their contract because they are angry should be connected with an agent skilled in retention and contract management with the highest priority. Additionally, lower priority or less urgent conversations can be held in the ‘bot’ as a holding pattern during times when service levels have exceeded a certain threshold. They can be offered self-service options or a call-back during times when service levels are exceeded. This makes room for the most impactful conversations that need immediate attention.
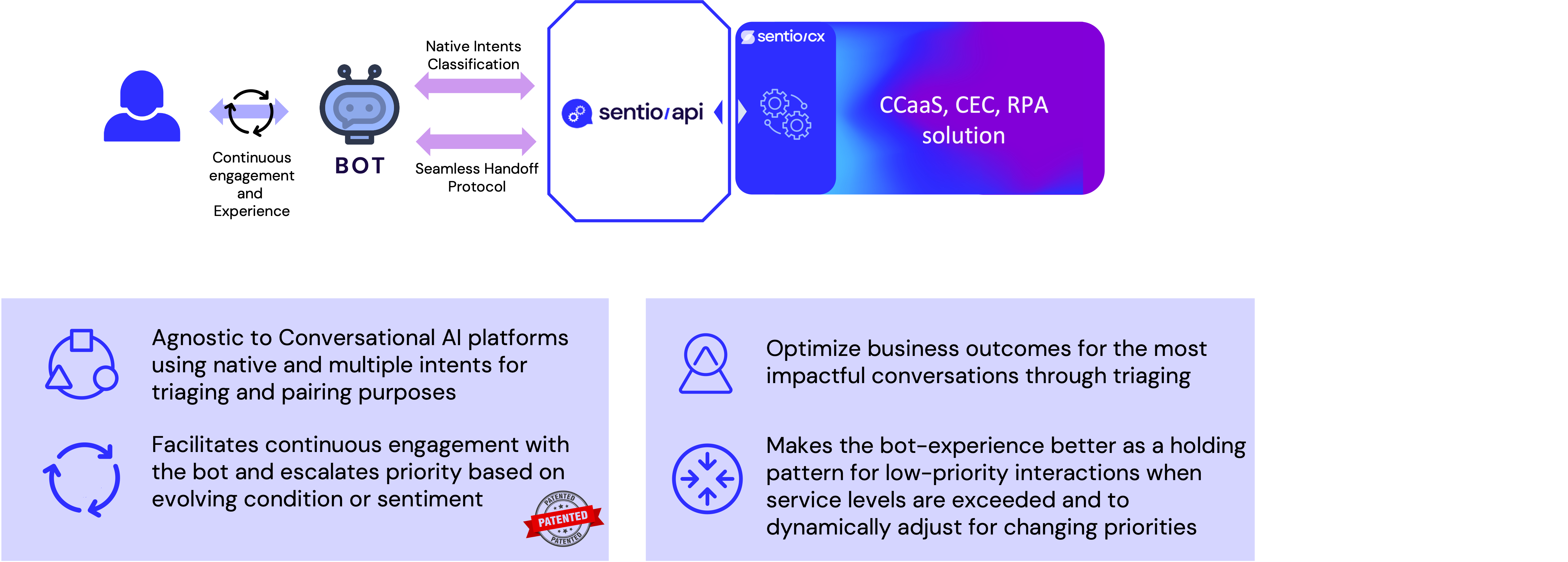
b. Solving the Complexity of Queue Management and SLA management
The complexity of queue management can be solved through continuous real-time triaging and automated SLA management, which reduces operational costs and improves both Customer Experience (CX) and Employee Experience (EX). Here is how it works:
When the system detects that service levels are being exceeded, it needs to create automated SLA reinforcement. Besides triaging of the most impactful conversations, additional agents should be notified when service levels are exceeded. We call these agents reserve agents or knowledge workers, as they are brought into the conversation when service level thresholds are exceeded or when specific skills and proficiency levels are required.
Lastly, when the agent pool remains insufficient to meet desired service levels, automatic deactivation of hand-off initiation should be allowed for selected (usually lower priority) conversations. This is a crucial part of our patented algorithm which is embedded into our SentioCX solution.
The following diagram depicts how it works:
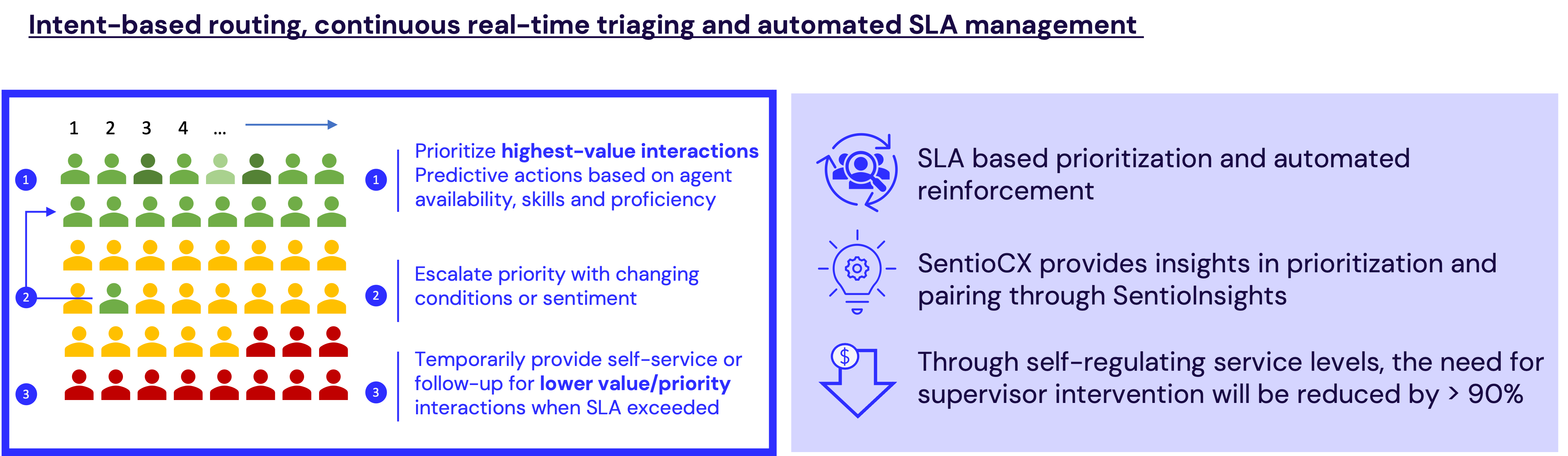
The net effect is that service levels are met for the most impactful conversations without penalizing lower-priority conversations. Additionally, by automating SLA management and applying self-regulating service levels, the need for supervisor intervention will be reduced by more than 90%.
Furthermore, instead of supervisors acting as ‘traffic police officers’ and reacting to circumstances such as angry customers, they should be able to be pro-active. This is done by having powerful dashboards to monitor service levels and adjusting the relevant orchestration parameters to allow the system itself to be hyper pro-active in determining Current Wait Time, Predictive Wait time and changing conditions including sentiment to self-regulate incoming conversations.
In 2023, we launched our first commercial release of SentioCX orchestration, based on our patented AI-enabled Intent-based Routing algorithm and the hard work of our talented development team. The solution includes the real-time continuous triaging and automated SLA management discussed in this blog post.
For a deeper-dive on our technology, please refer to our CTO Adrian Ivan’s blog or visit our development portal
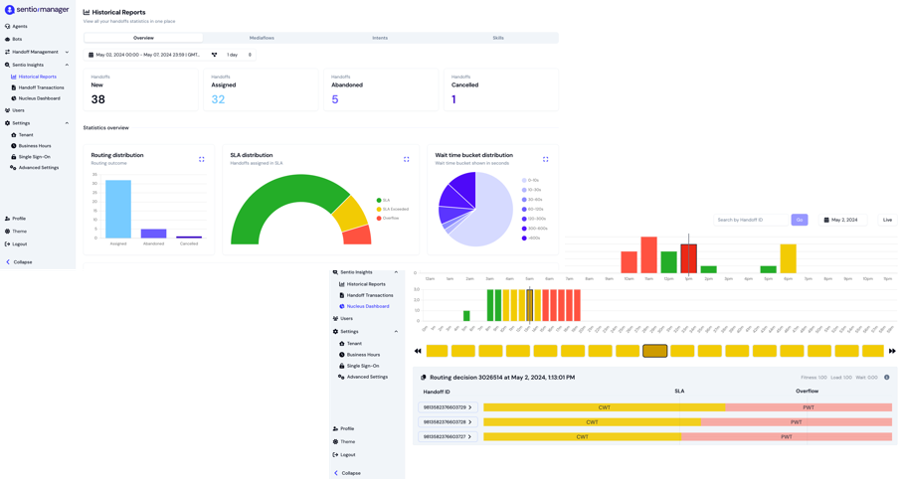
Queues are dead! Welcome AI-enabled Intent based Routing!
This article challenges the status quo in the Customer Experience (CX) industry regarding the usage of queues, arguing that they have become obsolete in modern business environments. In an era characterized by rapid technological advancements and evolving customer expectations, the traditional queue system no longer aligns with the need for seamless, efficient service delivery.
As businesses strive to optimize operational efficiency and enhance customer satisfaction, it’s imperative to explore alternatives that minimize wait times, reduce friction, and maximize productivity.
We’ll do a thorough examination of the limitations of queues and propose an innovative solution for a personalized customer experience that works seamlessly, with soon to be ubiquitous, chatbot technology. This holistic approach ensures that customers receive tailored assistance at every touchpoint, while also prioritizing measurable operational efficiency.
What is a Queue in Contact Center software?
A queue in Contact Center software is a prioritized line of interactions awaiting assignment to agents. Typically, the priority within the queue is determined by queuing time, following a First In First Out (FIFO) principle. However, in many cases, priority is based on specific business criteria such as customer profile or contact urgency, with FIFO used as a tiebreaker.
Contact Centers often utilize multiple queues, which may represent different priority tiers, customer support departments or areas of expertise.
Initially, a static triage is conducted to determine the appropriate queue and priority for each interaction. This triage occurs just once, typically based on information gathered during the IVR (Interactive Voice Response) or chatbot interaction.
Interaction routing
Routing entails the assignment of an agent to an interaction based on their capabilities, skills, availability, and capacity.
Agents are typically configured to handle interactions from multiple queues, leading to a scenario where interactions from different queues compete for the same pool of agents.
A simplified routing implementation involving multiple queues typically follows these steps:
• Interactions from all queues are aggregated into a global list.
• Interactions in the global list are ordered based on priority in the original queue.
• Interactions are assigned to available agents in priority order. This process considers factors such as the original queue-agent assignment, agent availability, capacity, and skills.
Queue operations
Supervisors establish performance targets, known as Service Level Agreements (SLAs), for queues. These SLAs typically include metrics like waiting time in queue or customer abandonment rate.
Supervisors continually monitor SLA compliance and enact corrective measures:
• Real-time adjustments involve reallocating agents to enhance throughput on queues experiencing issues.
• Historical analysis is conducted to identify improvement opportunities. This may involve allocating more agents, redesigning queues, enhancing individual agent performance, improving deflection strategies, and other relevant optimizations.
What are the challenges associated with Queues?
Queues have been around since Contact Centers have been invented and are the established strategy for prioritizing interactions and distributing work to agents.
Meanwhile, intelligent chatbots became mainstream and the focus in customer experience approach has shifted. The customer experience starts from the chatbot and extends into the Contact Center.
Queues were relevant in the IVR times, but what about the era of intelligent chatbots? Let’s find out.
Conceptual mismatch
At their core, queues are primarily designed for inward-facing work distribution (Work Queue) rather than as a means to enhance the customer experience.
There’s a clear need for an outward-facing, customer-oriented model. Customer experience design starts from the chatbot and extends into the Contact Center.
A dichotomy arises between the customer-oriented design of chatbots and the inward, task-oriented, nature of queues.
Insufficient personalization
Personalization in customer service ranks high among core Contact Center capabilities according to analyst reports. In the context of interaction routing, the objective is to swiftly pair customers with the most suitable agent by considering factors such as customer profile, interaction history, current needs, sentiment, and urgency.
However, queues primarily serve as a mechanism for ordering interactions, regulating traffic flow, and assigning work to agents.
Due to the coarse-grained nature of queuing, opportunities for personalized service become inherently limited once customers are placed in a queue. Nuanced customer needs are obscured, often resulting in a one-size-fits-all approach.
Triage
Queues-based systems traditionally offer a single opportunity for triage, occurring before interactions enter the queue. This static triage involves selecting the appropriate queue and, sometimes, overriding the default queue priority based on customer profile and custom rules. This model was suitable when IVRs guided customers to the appropriate queue.
However, with the widespread adoption of chatbots, customer expectations have changed. Customers now anticipate more engaging and empathetic interactions. Chatbots equipped with NLP features, including sentiment and urgency detection, have raised the bar for customer engagement. What if the Contact Center could leverage these sentiment and urgency fluctuations during a conversation to execute continuous and dynamic triage?
Dynamic triage is also desirable for internal stimuli, such as SLA monitoring events, allowing proactive corrective measures to be taken.
Using queues, stimuli cannot be dynamically incorporated into the process, limiting the system’s adaptability and responsiveness.
Penalized customers
In the rigid queuing model, low-priority customers often face significant penalization.
Lower-priority interactions are consistently overlooked, while high-priority ones continue to flood in.
But what if there was a prioritization model that balanced customer priority with wait time, ensuring everyone gets a fair chance?
Wait time expectations
Establishing clear customer waiting time expectations is a best practice in Contact Centers.
Some vendors offer predicted wait times, while others provide information on the customer’s position in queue.
However, in many cases, the estimated wait duration may not be accurate. For instance, the position in a queue may not hold much relevance if agents handle work from multiple queues simultaneously. Similarly, predicted wait times computed based on queue throughput statistics may lack precision due to factors like agent churn and agents’ involvement in multiple queues.
Queue design strategy
The design strategy for queues typically revolves around criteria such as priority, business topic or agent department.
However, in complex customer support organizations, achieving desired outcomes can be challenging due to the coarse-grained nature of queues. This often leads to compromises:
Priority Queues:
• The context of customer issues may be diluted.
• Implementing continuous improvement strategies based on irrelevant historical reports can be difficult.
• More suitable for simple organizations with a limited number of defined priorities.
Business Topic Queues:
• Customer profiles are not leveraged effectively.
• Maintaining triage rules to enhance customer affinity can be challenging.
• SLAs based on customer importance cannot be implemented.
• Queues number explosion, where the number of queues becomes unmanageable.
Department Queues:
• The design is rigid due to the limited number and siloed nature of departments in the organization.
Skills based Routing is implemented to alleviate certain challenges, but introduces its own set of challenges:
• It’s unclear which routing strategy takes precedence, queueing or skills-based.
• Monitoring, reporting, and analytics are typically based on queues, limiting the effective application of skills-based routing.
Operational burden
Supervisors monitor queues and intervene manually when SLAs are not met.
Usually, supervisors resort to manually reallocating agents between queues to improve the situation.
This manual process relies heavily on human expertise and is characterized by reduced predictability and repeatability.
A more effective approach is to declaratively configure the desired system behavior when operational quality decreases.
Root cause analysis
In real-time scenarios, supervisors must promptly identify issues and their root causes. However, queues often lack the precision required to take the best corrective actions.
Continuous improvement in customer experience is essential. Supervisors analyze historical data to identify areas for improvement. However, queue-level reporting often fails to provide sufficient insight into actual customer pain points, hindering the implementation of specific improvement measures.
Is there a better alternative to Queues?
Absolutely! The challenges associated with queues can be summarized into these key issues:
• Granularity: Queues lack the granularity needed for customer affinity, personalization, and effective triage.
• Rigidity: Queues imply a fixed interaction ordering after the initial triage, limiting flexibility.
• Worker-oriented: The focus is on emptying queues quickly rather than providing personalized service.
• Inward-oriented: Modern customer experience design begins with the chatbot and extends to the Contact Center. Queues, which worked well with IVR systems collecting simple information, are not suitable for a qualitative end-to-end customer experience.
An alternative approach is needed to address these shortcomings and provide a more dynamic and customer-centric solution.
What does a better alternative look like?
• Identifying fine-grained concepts that capture customer needs and utilizing them to construct an end-to-end personalized customer experience, beginning from the chatbot and extending to routing.
• Ability to perform dynamic triage, allowing for real-time adjustments in priority and routing requirements.
• Implementing a flexible prioritization algorithm that balances the influence of nominal interaction priority and wait time.
• Empowering supervisors with detailed and relevant insights based on actual customer needs, facilitating continuous improvement opportunities.
• Enabling proactive and automated SLA enforcement, granting supervisors the capability to automate system behavior when SLAs are not met, ensuring consistent service quality and predictability.
AI-enabled Intent based Routing
Introducing AI-enabled Intent based Routing!
This cutting-edge approach harnesses real-time customer intent, sentiment, urgency, and historical profiles to seamlessly match interactions with the most suitable agents at the optimal moment.
Intent based
Intent serves as the granular representation of customer needs, answering the fundamental question: Why is the customer engaging with us now?
It offers unparalleled alignment with the customer’s query, facilitating personalized interactions.
Utilizing NLU/NLP chatbot capabilities, AI-driven intents direct the routing process.
Dynamic
Unlike static queueing systems, our router dynamically triages interactions in real-time, responding to external and internal triggers such as changes in intent, sentiment, urgency, and SLA monitoring events. This ensures interactions are prioritized dynamically, enhancing responsiveness and adaptability.
Intelligent
Our algorithm employs a sophisticated approach to prioritize interactions, blending traditional criteria like waiting time and customer profiles with real-time conversation insights such as intent, urgency, and sentiment. By utilizing a flexible formula for priority scoring, our ranking algorithm delivers equitable service across the board, without compromising on efficiency.
Routing
Our router adopts a skills-based routing strategy, aligning agent skills with interaction intent. This strategy maximizes effectiveness, ensuring interactions are routed to agents with the highest affinity, ultimately enhancing the overall customer experience.
Tunable and transparent
Supervisors have access to sophisticated tuning knobs, ensuring routing behavior is tailored to specific business needs. Supervisors act guided by rich real-time and historical insights.
How does it work?
Queues revisited

• Customers initially engage with the Contact Center through IVR scripts.
• Escalated interactions undergo a single triage process and are then sorted into priority queues (static triage).
• The overall priority order dictates that high-priority interactions are prioritized for assignment ahead of others.
• Lower-priority interactions remain in queue, waiting until high-priority interactions have been addressed.
AI-enabled Intent based Routing
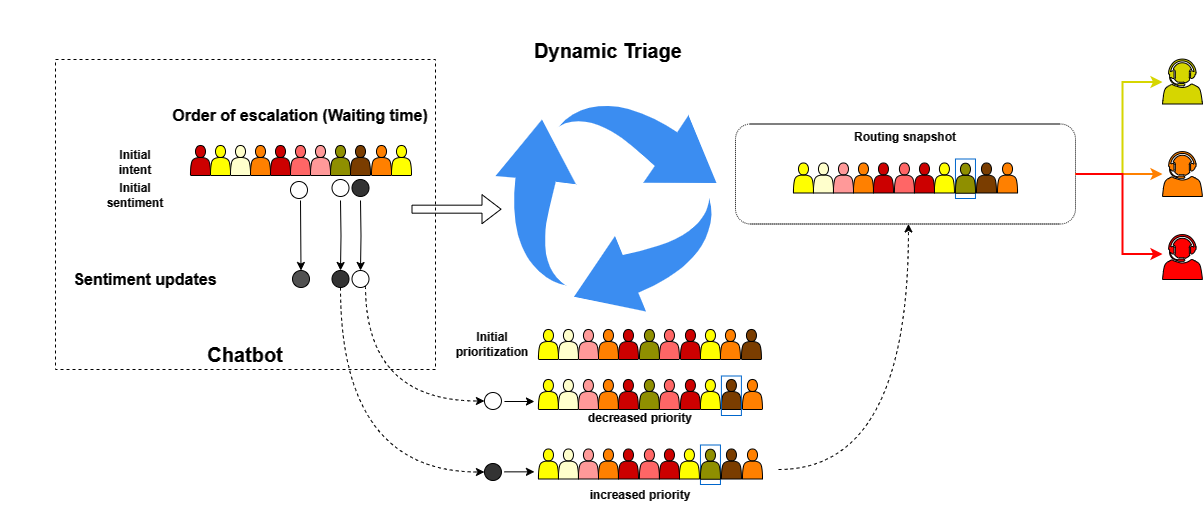
• Customers engage with the Contact Center through Chatbot conversations.|
• Escalated interactions undergo dynamic triage, which adapts in response to changes in intent, sentiment, and urgency.
• The prioritization of interactions blends factors such as intent significance, customer sentiment, urgency, and current wait time, ensuring equitable allocation for lower-priority interactions.
• Dynamically triaged interactions are then paired with the most suitable agents, aligning their skillset and proficiency with the specific requirements outlined by the customer’s intent.
The picture provides an example where interactions are dynamically triaged based on customer sentiment updates:
• the initial prioritization considers the customer intent, sentiment, profile and wait time
the sentiment fluctuations lead to reprioritization of interactions
What are the benefits?
Holistic Customer Experience design
The design of the chatbot experience is rooted in intent detection, a foundation that seamlessly extends to the triage and routing processes.
This integrated approach bridges the gap between the chatbot and Contact Center realms, ensuring a cohesive customer journey without the disjointed “throw over the fence” experience. By utilizing consistent intents throughout, customers benefit from a unified and intuitive interaction regardless of the channel they engage with, fostering a seamless and cohesive experience.
Personalization
Prioritization
Unlike queue, intents provide higher precision for classifying interactions.
Interaction priority considers customer intent, sentiment and urgency, providing improved customer affinity in the support process.
Best Agent
Intents offer a detailed method for identifying the specific business case and selecting the most suitable agents to address customer needs.
Aligning intents with the necessary agent skills allows for the best implementation of a Skills-Based Routing strategy. This approach establishes a direct connection between the customer’s actual requirements and the relevant skill set of the agents. Unlike queueing systems, which may involve intermediate steps, this strategy ensures that agent skills remain undiluted and directly applicable to customer needs.
Dynamic triage
Interactions undergo dynamic triage, leading to a prioritization that accurately mirrors the real-time customer experience.
This triage process has the capability to adjust interaction priorities, either elevating or lowering them based on the evolving circumstances. In instances where Service Level Agreements (SLAs) are not met, customers may even be redirected back to the chatbot for further assistance.
Improved KPIs
Fine-grained intents, paired with Skills-based Routing, contribute to decreased handling times (AHT) and enhanced first call resolution (FCR), resulting in heightened customer satisfaction and boosted agent morale.
Customer fairness
As the wait time grows, the ranking algorithm ensures that low-priority interactions have an real opportunity to be assigned to agents.
The supervisors may fine-tune the algorithm parameters to increase or decrease the chance according to the business context.
Opportunity to pair
The most impactful interactions should be handled by the best agents. Without a fixed order queueing system, the router can reserve available agents for potentially more impactful interactions.
Consequently, less impactful interactions may experience a delay. Supervisors possess the tools to adjust and refine this behavior according to specific needs.
Accurate Predictive Wait Time
The routing engine offers a predicted wait time derived from agent-level statistics, enabling the anticipation of the most suitable agent and the timing of pairing.
Operations automation
Real-time routing statistics at the intent level and SLA monitoring enable a transformative shift in Contact Center operations.
Contact Centers currently operate under manual models reminiscent of pre-Cloud Computing practices, where software deployment and management were manually handled in data centers.
In a Cloud native metaphor:
• Throttling and rate limiting: Interactions for low-priority intents are de-prioritized and routed back to the chatbot, as configured by supervisors.
• Elasticity: The system dynamically scales out by adding additional resources when SLAs are not met; on-call agents are automatically mobilized to handle increased workload.
Insights and root cause analysis
Intents serve as valuable tools for conducting effective insights and root cause analysis.
By offering insights at the intent level, supervisors can swiftly identify areas requiring improvement during performance evaluations.
The analytics engine furnishes intent and skills-level statistics pertaining to interaction routing outcomes and customer experiences. This data enables thorough analysis and informed decision-making to enhance overall operations and customer satisfaction.
Comparison table
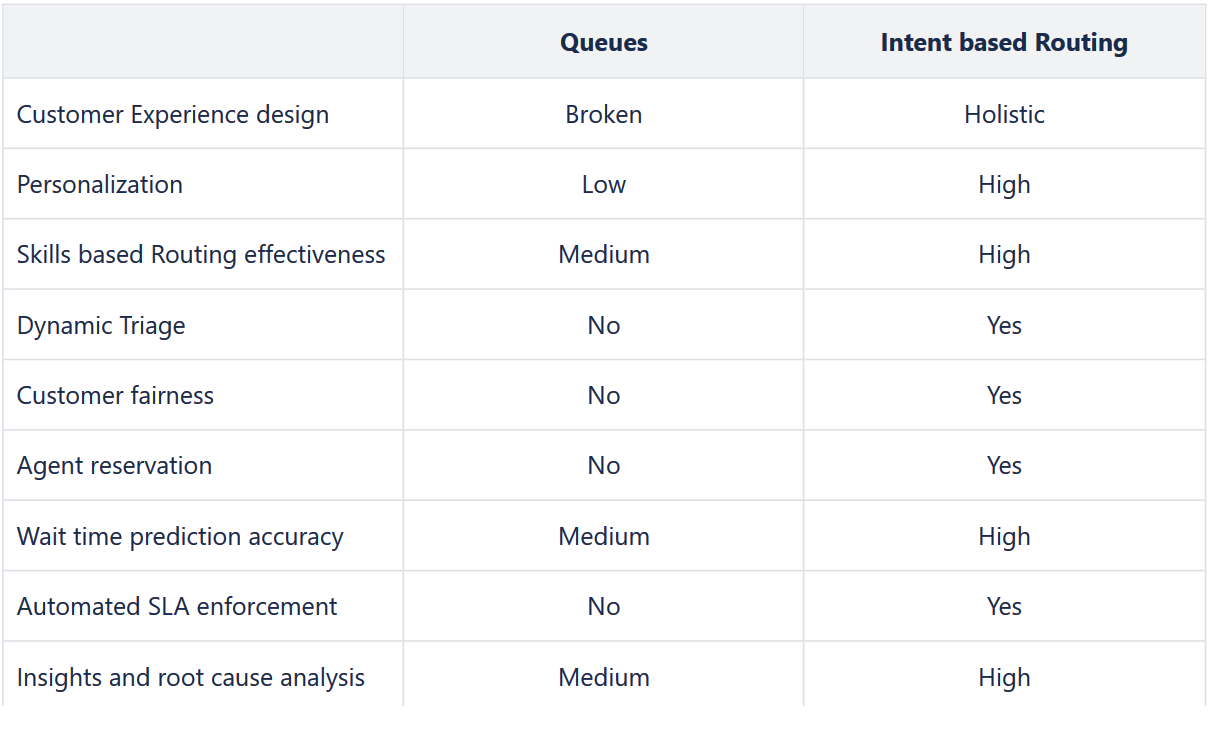
Closing thoughts
Using queues, stimuli cannot be dynamically incorporated into the process, limiting the system’s adaptability and responsiveness.
AI-enabled Intent based Routing is available in the SentioCX Conversation Orchestration platform. Please visit us at www.sentiocx.com.
Why Conversation Orchestration is essential to great AI driven customer service
Since the beginning of this year, barely a day has passed without AI and ChatGPT grabbing headline news. These technologies are profoundly and permanently impacting our society. In this blog, we’re eager to share our insights on how the rapid adoption of AI and ChatGPT is changing the traditional Call Center and CCaaS (Contact Center as a Service) industry. We will elaborate on the 5 reasons why conversation orchestration is essential by facilitating an effective Human-In-The-Loop (HITL) as the last mile to make the customer experience seamless and frictionless.
1. AI and ChatGPT are powering the next big leap in customer self-service.
Conversational AI platforms are catalyzing a significant transformation in customer self-service. These technologies offer vast enhancements over the previous generation of chatbots, which previously frustrated customers with misunderstandings and irrelevant responses. The new generation of chatbots, powered by AI and ChatGPT, as a subset of Large Language Models (LLMs), excels in generating human-like responses that effectively address a broad spectrum of customer queries. These advanced chatbots can provide tailored responses to meet specific customer needs, leading to shorter wait times and enhanced overall satisfaction. They possess the capability to learn autonomously from past conversations, equipping them with extensive knowledge from data sources. As such, it is no longer a case of if CX organizations will be forced to further invest in conversational AI, but when. Ensuring that this new wave of technology is properly architected to deliver for customers is essential.
2. Digital self-solutions are not yet delivering their full promise.
Customer Service executives are confronting a perfect storm of challenges, including increased call volumes and a talent shortage. Add to this mix inflated customer expectations and high-effort service designs, and it becomes easy to understand why they are under significant pressure.
Digital and Customer Self-service solutions have emerged as an increasingly prevalent tool to address customer service challenges and to optimize operational efficiency. CX Leaders often perceive these technologies as a win-win, offering cost savings and delivering a great experience through quick resolutions. There is research to back this – with Salesforce’s 2023 state of Customer Service suggesting that 59% of customers even prefer self-service tools for simple questions/issues.
So, what’s the problem you may ask? With 88% of customer journeys starting in self-service touching multiple channels according to the latest survey of Gartner in December 2022, only a shocking 13% of customers are fully able to resolve their issues through self-service as the only channel.
Gartner
The same survey of 1,492 B2B and B2C customers found that a seamless transition between channels is a key driver of positive customer experience (CX) outcomes and future self-service adoption. When the transition is high effort, meaning that switching channels did not lead to resolution, the probability of positive CX and loyalty outcomes is dismal. Conversely, 74% of customers who experience easy transitions to a customer service representative say they’ll return to self-service next time. Ensuring an easy transition between channels, and particularly to a live agent, is critical to deliver on the value of self-service/AI for both customers and businesses.
“Despite resolving their issue in assisted service, these customers are just as likely to use self-service in the future as those whose issues were fully resolved using self-service. Eric Keller, Sr. Director Analyst, Gartner”
3. Effortless Transitions Improve Business KPI’s and Customer Experience.
The above survey highlights the benefits of promoting seamless transitions between self-service and assisted channels. Seamless transitions improve Customer Experience across a range of metrics and boost loyalty, but they also save time and resources. In fact, 93% of respondents reported high customer satisfaction (CSAT) when there was a seamless channel transition.
4. Human-In-The-Loop (HITL): Desired or Required When It Matters
When designing customer self-service, we should treat the Human-In-The-Loop (HITL) as the last-mile to make the customer service experience seamless and frictionless.
In short, there are several occasions where we need to bring in the knowledge, flexibility and skills that only a human agent or knowledge worker can deliver:
a) Complicated issues where self-service is not able to drive the issue to resolution.
When customers need to modify their contracts, they often require the expertise of a live agent knowledgeable in contract management and legal matters. Instances where a bot might ask too many clarifying questions, leading to customer annoyance, further highlight the need for human intervention. Additionally, complex issues, particularly in banking or other specialized areas, require support of knowledgeable professionals for the foreseeable future.
b) When detecting a buying or a defecting customer signal
Companies keen on not missing revenue opportunities must be adept at identifying the right moments for a live agent to step in—especially when a customer shows interest in making a purchase or exhibits signs of potential departure. In such instances, it’s crucial for the bot to recognize these signals promptly and insert the most relevant salesperson or expert with the highest priority through real-time triaging.
c) Human Empathy
Early detection of customer sentiment allows businesses to capture their customers’ feelings and respond appropriately via live agents. There’s a common belief that bots lack empathy and that demonstrating empathy effectively requires promptly connecting customers to a human agent. However, some staunch technologists argue that achieving empathetic responses through technology is only a matter of time. Our research indicates that customers tend to become more irritated with perceived ‘fake empathy’ from a bot than when interacting with a human being. Therefore, ensuring a seamless transition to a live agent when it truly matters is crucial.”
Take, for example, an insurance company in the Netherlands that has been utilizing conversational AI with success. Despite customer satisfaction with the bot, the company occasionally routes customers to live agents as a gesture of care. This inclusion of a human touchpoint, even briefly, reinforces customer care before handing back the conversation to the bot.
So, Bots aren’t good at delivering empathy to customers, but they’re increasingly good at understanding how the customer feels. You don’t want the AI to deliver the empathy, you want to leverage the AI to both prime the agent to know the ideal tone to set, and to pick the agent best able to deliver that as well.
In an era of advanced AI and ChatGPT, customers expect immediate and accurate responses. Yet, many existing technologies only provide basic First-In-First-Out or are leveraging multiple queues in traditional call centers as hand-offs from bots to human agents without any ability to manage traffic, prioritize crucial conversations, or seamlessly connect users with specialized knowledge workers. This erratic transfer process often results in the frustration of unpredictable waiting times and in some cases agents who are unable to resolve issues within the first contact. As a result, the existing disconnect between bots and human agents is becoming increasingly undesirable in today’s customer-centric landscape. Providing the opportunity for a simple escape from a bot to a live agent is essential. This fact has led to the necessity of what we now call conversation orchestration. In an ideal world, perfect harmony should exist among digital self-service channels and live agents or knowledge workers for an optimized customer experience.
5. Conversation Orchestration: the missing piece of the puzzle in AI enhance Customer Experience?
In addition to its application in conversational AI, Generative AI also plays a significant role in AI Agent Assist, a technology that supports human agents during customer interactions. By providing real-time suggestions and relevant information, ChatGPT can help agents respond more effectively and efficiently to customer inquiries. However, what Conversational AI and AI enabled Agent Assist are not resolving is the absence of harmony between automated responses and live agent interaction.
Here at SentioCX, we envision a future where transitioning conversations from digital self-service solutions to live agents is effortless and seamless. The future of customer service will involve chatbots and live agents working together in harmony to provide the best outcome to customers and agents. Conversational Orchestration leverages AI to facilitate agent-pairing and to orchestrate conversations. This enables organizations to take advantage of conversational AI and operational efficiency while also optimizing customer intimacy. In Ronald’s previous blog “Resolving the Customer Experience paradox”, the point was made that current routing algorithms in CCaaS solutions are outdated. They cannot make routing decisions based on recognition of real time intents or sentiment from the bot. Hence, we came up with a new way of revolutionizing Human-In-The-Loop. We use AI based agent pairing to create seamless and effortless transitions from digital self-service to live agents and knowledge workers.
The essential components of AI enhanced Customer Experience
By using the three pillars of AI enhanced Customer Experience combined with AI and ChatGPT, we provide an evolution on the now obsolete traditional IVR. Users can effortlessly ask questions and receive automated responses or when necessary be connected directly to the right agent or knowledge worker to solve their issue.
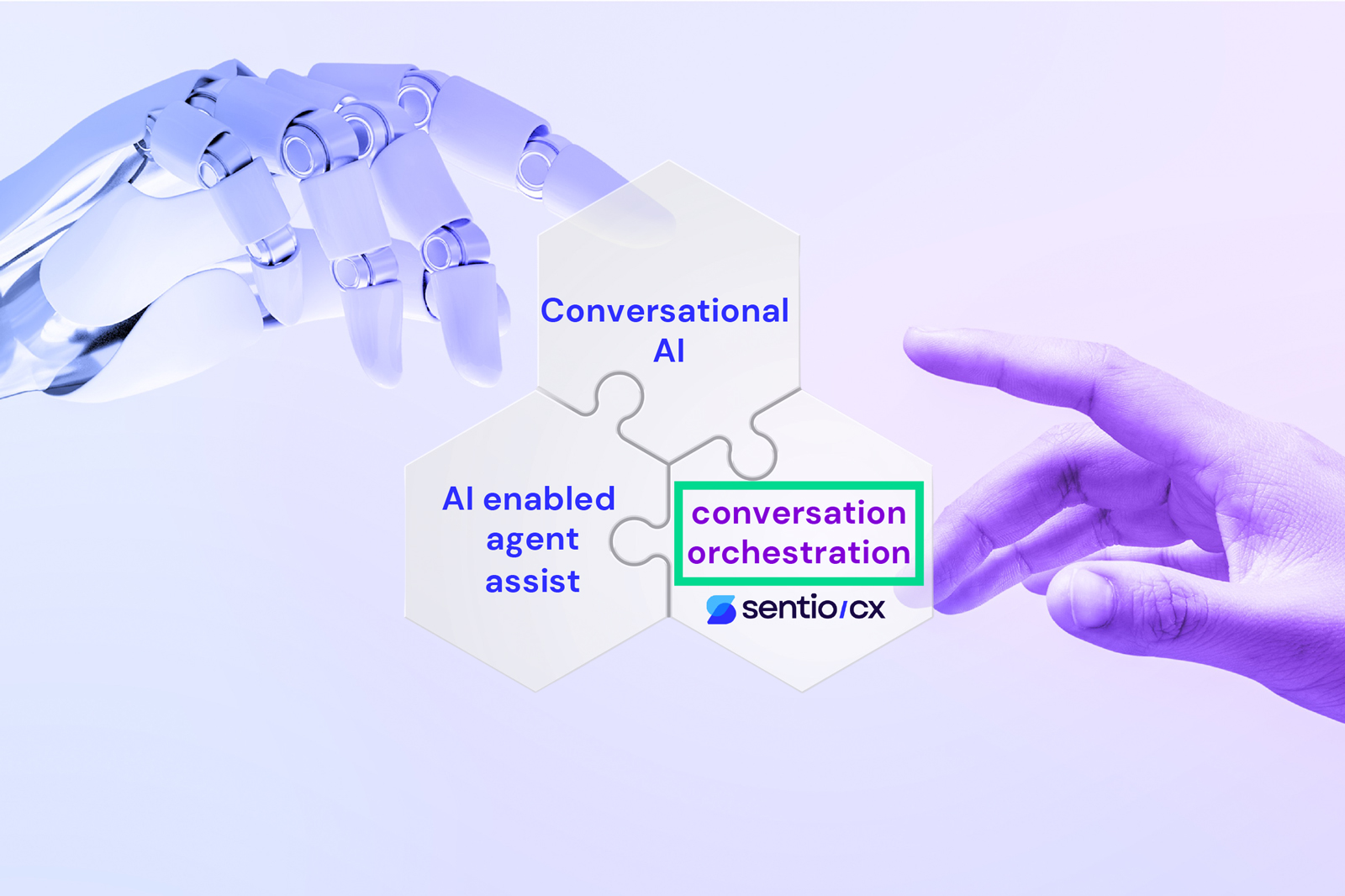
In summary
Conversation Orchestration effectively prioritizes high-value interactions, through predictive actions based on agent availability, skills, and proficiency. Furthermore, it dynamically escalates priority with changing conditions or sentiment and temporarily provides self-service or follow-up for lower value/priority interactions when service levels are exceeded. This approach is designed to optimize the impact on key performance indicators (KPIs) such as customer churn, customer satisfaction, and (up and cross) sales. SentioCX delivers these capabilities out of the box along with dashboards to manage, track and visualize the impact on the most relevant KPIs.
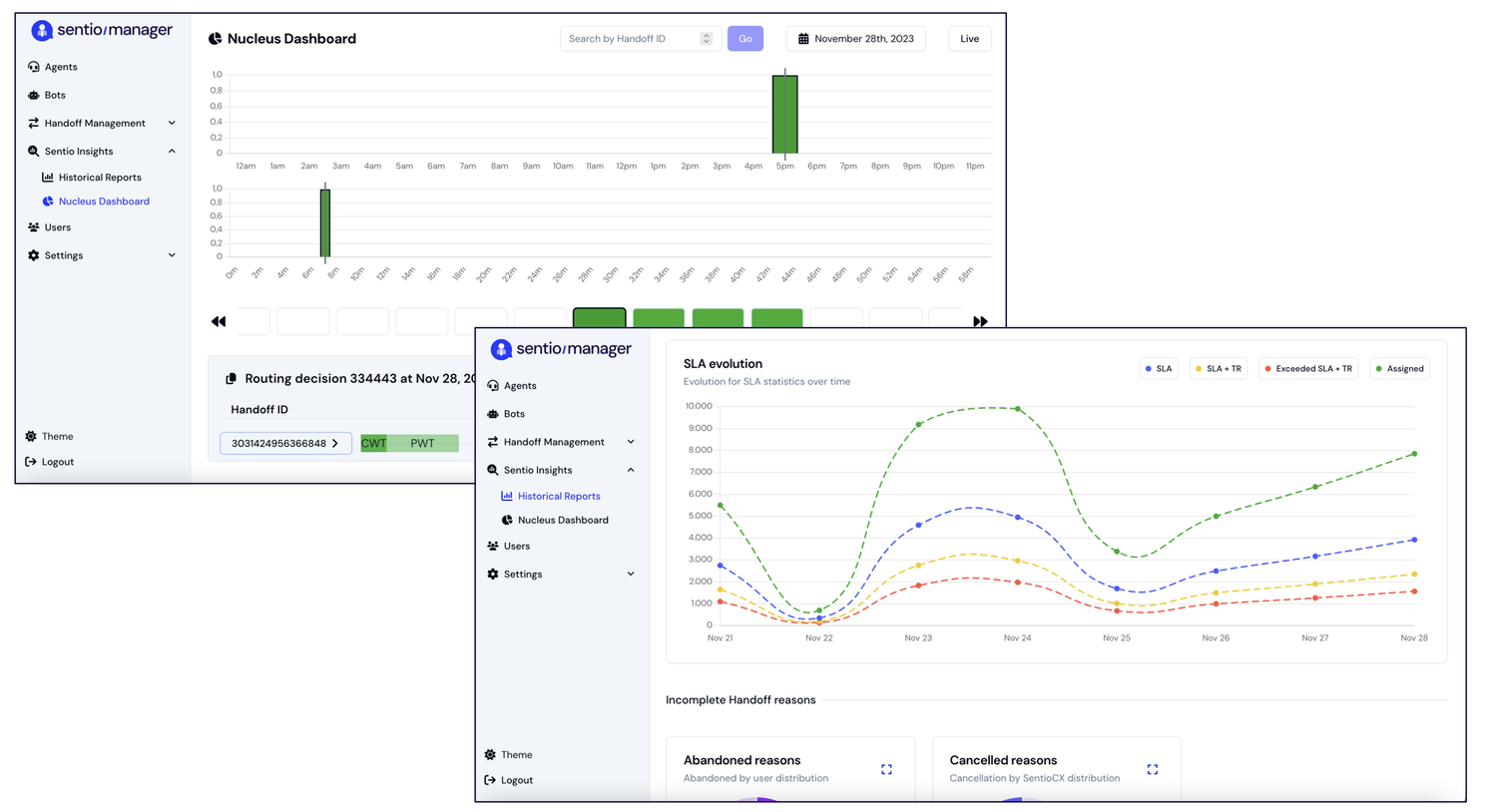
Sentio Insights provides dashboards and reports to manage, track and visualize KPIs and Service Levels
Resolving the Customer Experience paradox
Ronald Rubens
My experience in the world of Customer Experience over the last 25 years
by: Ronald Rubens
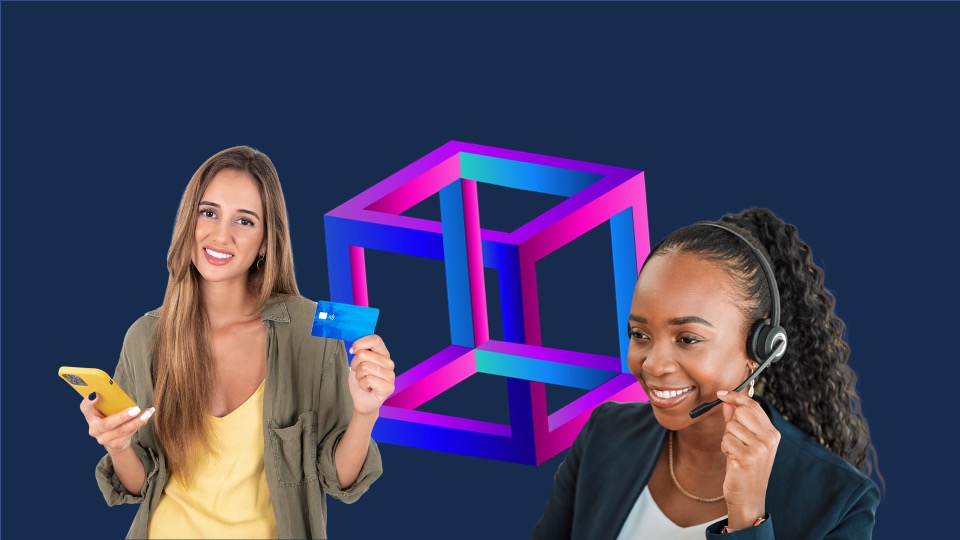
Today are both exciting and alarming times in the world of customer experience. Over the last 25 years, I have seen how new technologies can create extra-ordinary experiences for customers and organizations. However, the reality on the ground is often different as organizations in their quest for optimizing operational efficiency, have applied a variety of technologies, such as bots, to take over where skilled people and knowledge workers once excelled. These bots turned out to be effective by successfully managing customer interactions, but also created friction or revenue loss when customers expected immediate access to a live agent. The good news is that I believe this old-age paradox of customer excellence versus operational efficiency is finally behind us; if organizations are doing the right things, they can now create extra-ordinary experiences while benefiting from operational efficiencies. However, before we get to the answer on how to achieve this, let’s have a look at some current facts and allow me to take you back in history.
It was only the other day that I had to wait for more than 24 hours to get an answer from a major airline on an urgent WhatsApp request, impacting an important customer visit. The next day I had to contact my energy company to renew a contract, but could not find the energy company’s phone number on their website. Given the recent developments on energy, the energy company took the decision to hide their customer service phone numbers with the hope that most interactions could now be taken over by a bot. And speaking about bots, who has not recently interacted with a bot to find out that the bot didn’t understand our question or was not able to transfer us to an agent? Or when the bot finally agreed to transfer us to an agent, we had to repeat the information all over again or found out that the customer service agent was not the right person to help us out.
It seems that I was not alone in these challenges. Hold-times in call center queues have increased by more than 50% over the last two years. Despite attempts to reduce hold-times by using self-service capabilities, such as ‘interactive voice response’ and bots, 59% of all consumers feel companies have lost touch with the human element of customer experience. In a recent survey by Zendesk, 54% of respondents say it takes too many questions for the bot to recognize it can’t answer the issue and then they must start all over with a human agent. As a result of these recent experiences 61% of customers would now defect to a competitor after just one bad experience as expectations around customer experience have risen in the digital age. So, what can we learn from history?
At the turn of the century, I worked in the United States with a talented group of Bell Labs Engineers in the contact center division of what is now called Avaya. My manager at the time gave me a book titled: “The Discipline of Market Leaders” by Treacy and Wiersema. It was one of these New York Time best sellers, which instantly became a success as it described three paths to market leadership. These three paths – also known as value disciplines – were customer intimacy, operational excellence, and product leadership. In essence, a company had to pick one of these disciplines while meeting industry standards in the other two. To become ‘Masters of two’ of these disciplines was a real challenge as inherent tensions would appear between the operating models that each value discipline demanded. In the contact center and customer experience space, this led to a so-called customer experience paradox as customer intimacy and operational efficiency were hard to unite under a single all-encompassing value discipline. Companies had to deal with trade-offs between the quality of the customer experience and the costs of providing it. This trade-off always seemed baffling to me, and a life-long quest started to explore how we could break this paradox and to bring both customer intimacy and operational excellence in balance.
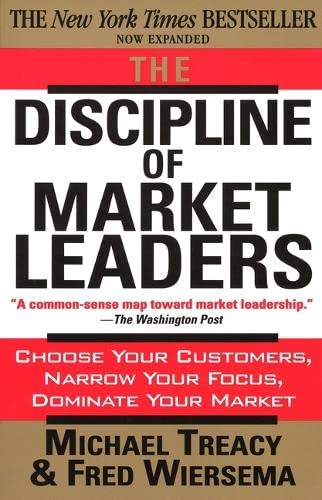
Twenty years later, encouraged and inspired with the famous words of Steve Jobs: “You’ve got to start with the customer experience and work your way backwards to the technology”, I started a discovery of the fundamental issues in the customer experience space and founded what is now SentioCX. “Sentio” is a Latin word for “I experience” or “I feel” to emphasize the starting point of customer experience. Our vision was to find a way to have technology – in this case bots – and humans work together seamlessly, to create extra-ordinary and frictionless experiences for the customer and as a result create an optimum between operational efficiency and customer intimacy. Today, we believe that we are finally able to unite these two paradoxical disciplines.
Our vision at SentioCX was to have companies, who were using bots and conversational AI technology, benefit from cost savings while at the same time improving customer retention and satisfaction by providing a seamless transfer from a bot to a live agent. It seemed simple and straightforward as I write this, but the challenges to make this happen were multiple. How do we make it seamless for customers? How can we ensure that questions from customers get solved the first-time? When is the right moment for the bot to hand-off the call to a live agent – in some cases pro-actively? How do we make sure the most qualified agent gets a specific call? The proliferation of Artificial Intelligence and a variety of omni channel concepts brought many powerful use-cases for Contact-Center-as-a-Service and Conversational AI technology, except providing a seamless transfer from a bot to a live agent. At best, agent hand-offs from a bot were ‘blind transfers’ by throwing a ball over the fence or launching chats in agent queues with the hope that the agents would pick out the most relevant chats. So, what was the answer?
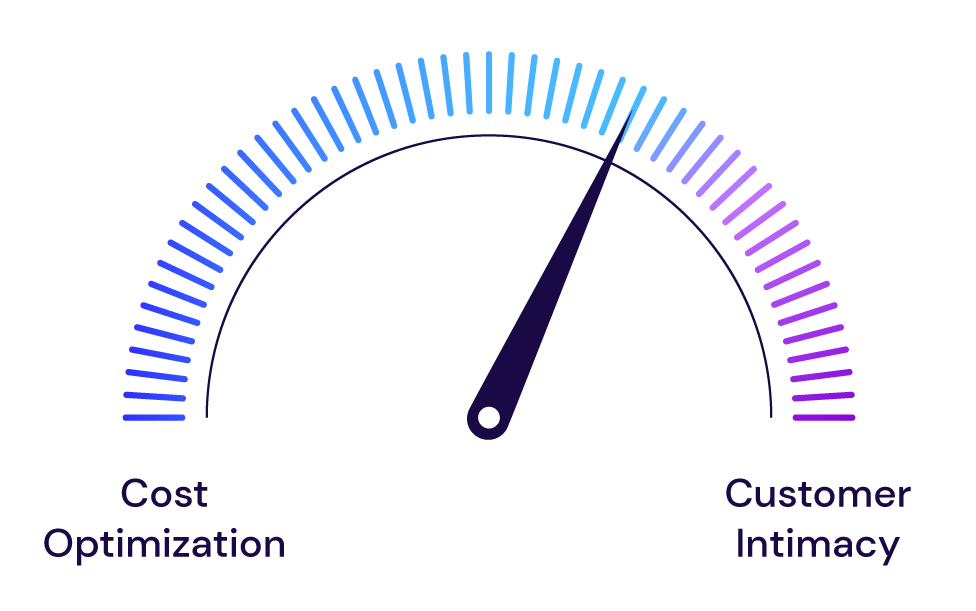
Along with deep integration with bots, conversational AI applications, service management and live chat applications, we discovered very early on that we needed ‘a routing algorithm’. After doing extensive research of ACD (Automatic Call Distribution) routing algorithms known today in the contact center and CCaaS (Contact-Center-as-a-Service) space, we discovered that most routing algorithms were too basic in the sense that they could only route chats or voice calls based on historical importance of the customer (retrieved from a CRM system) or IVR (Interactive Voice Response) choices. Hence, we couldn’t use these existing algorithms to facilitate a seamless hand-off to an agent.
The customer experience we wanted to provide was that by applying Artificial Intelligence, the bot would understand the customer and more importantly, detects ‘early on’ that it doesn’t understand the customer or believes that human intervention is required. It would then pro-actively with priority and/or urgency seamlessly hand-off the chat to the right agent with the right skills and proficiency levels or if necessary to a knowledge worker with a specialized skill.
What we wanted to accomplish was apart from the customer profile, to classify and prioritize real time intents captured by the bot and based on these intents match the chat with an agent or knowledge worker with the relevant skills and proficiency levels and dynamically adjust the routing priority. So, what we did was to start inventing a ‘brand new approach’ of a sophisticated ACD (Automatic Call Distribution), which we now refer to as our ‘Intent-based Intelligent Routing engine’. And so it began: we filed a patent, simulated the algorithm, validated it, and started to build the product by hiring a bunch of talented software engineers who were as passionate about this business as we were.
During the time of Tracey and Wiersema’s publication of their book, customer experience managers had a challenging job: they had to choose between operational efficiency and customer experience. Today we believe that with the advent of technology, and our work at SentioCX, we are now able to unite these two paradoxical disciplines. I am excited about the future. Not only for our company and the difference we are making, but also for the future of the CX industry and the end-customer. I believe that with the right actions, we can create a perfect symbiose of technology, bots, call center agents and knowledge workers, working to create great experiences for customers and organizations.